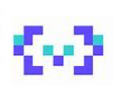
AgentScope Learning Feedback
│|├── rpc# Rpc模块,用于智能体分布式部署。│|├── message.py# 智能体之间消息传递的定义和实现。│|├── agents# 与智能体相关的核心组件和实现。│|├── models# 用于集成不同模型API的接口。│|├── memory# 智能体记忆相关的结构。
教程:关于AgentScope — AgentScope 文档 (modelscope.github.io)
AgentScope代码结构
AgentScope
├── src
│ ├── agentscope
│ | ├── agents # 与智能体相关的核心组件和实现。
│ | ├── memory # 智能体记忆相关的结构。
│ | ├── models # 用于集成不同模型API的接口。
│ | ├── pipeline # 基础组件和实现,用于运行工作流。
│ | ├── rpc # Rpc模块,用于智能体分布式部署。
│ | ├── service # 为智能体提供各种功能的服务。
| | ├── web # 基于网页的用户交互界面。
│ | ├── utils # 辅助工具和帮助函数。
│ | ├── prompt.py # 提示工程模块。
│ | ├── message.py # 智能体之间消息传递的定义和实现。
│ | ├── ... ..
│ | ├── ... ..
├── scripts # 用于启动本地模型API的脚本。
├── examples # 不同应用程序的预构建示例。
├── docs # 教程和API参考文档。
├── tests # 单元测试模块,用于持续集成。
├── LICENSE # AgentScope使用的官方许可协议。
└── setup.py # 用于安装的设置脚本。
├── ... ..
└── ... ..
示例解读
Quick Start对话
# -*- coding: utf-8 -*-
"""A simple example for auto discussion: the agent builder automatically\
set up the agents participating the discussion ."""
from tools import load_txt, extract_scenario_and_participants
import agentscope
from agentscope.agents import DialogAgent
from agentscope.pipelines.functional import sequentialpipeline
from agentscope.message import Msg
model_configs = [
{
"model_type": "openai",
"config_name": "gpt-3.5-turbo",
"model": "gpt-3.5-turbo",
"api_key": "xxx", # Load from env if not provided
"organization": "xxx", # Load from env if not provided
"generate_args": {
"temperature": 0.5,
},
},
{
"model_type": "post_api_chat",
"config_name": "my_post_api",
"api_url": "https://xxx",
"headers": {},
"json_args": {},
},
]
agentscope.init(model_configs=model_configs)
# init agent_builder
agent_builder = DialogAgent(
name="agent_builder",
sys_prompt="You're a helpful assistant.",
model_config_name="my_post_api",
)
max_round = 2
query = "Say the pupil of your eye has a diameter of 5 mm and you have a \
telescope with an aperture of 50 cm. How much more light can the \
telescope gather than your eye?"
# get the discussion scenario and participant agents
x = load_txt("agent_builder_instruct.txt").format(
question=query,
)
x = Msg("user", x)
settings = agent_builder(x)
scenario_participants = extract_scenario_and_participants(settings["content"])
# set the agents that participant the discussion
agents = [
DialogAgent(
name=key,
sys_prompt=val,
model_config_name="my_post_api",
)
for key, val in scenario_participants["Participants"].items()
]
# begin discussion
msg = Msg("user", f"let's discuss to solve the question: {query}")
for i in range(max_round):
msg = sequentialpipeline(agents, msg)
(1)配置接口
(2)创建一个Agent,被设置为使用my_post_api
(3)把用户的问题也就是query放入xt文件中的prompt
(4)打包成Message丢给agent_bulider
(5)extract_scenario_and_participants 的作用是从 agent_builder 的响应中提取对话场景和参与者信息
(6)根据场景和参与者信息,创建一个对话代理列表 agents。
(7)最后对话由用户发起,通过 Msg 类创建一个用户消息(msg),然后使用 sequentialpipeline 函数进行迭代,每个代理按顺序处理消息,直到达到最大轮次或解决问题。
个人感想:貌似比MetaGPT的框架要更直白简介一些
遇到的小问题
OpenAI的用不了,还是比较喜欢国内的大模型API
qwen配置:
{
"model_type": "tongyi_chat",
"config_name": "qwen",
"model_name": "qwen-72b-chat", # 72b,max都限时免费
"api_key": "Your API KEY" # 放自己的API KEY
}
examples文件夹中的代码这部分写了个"model",应该改成"model_name"才会被识别
狼人杀
HostMsg = partial(Msg, name="Moderator", echo=True)
healing, poison = True, True
MAX_WEREWOLF_DISCUSSION_ROUND = 3
MAX_GAME_ROUND = 6
# read model and agent configs, and initialize agents automatically
survivors = agentscope.init(
model_configs="./configs/model_configs.json",
agent_configs="./configs/agent_configs.json",
)
roles = ["werewolf", "werewolf", "villager", "villager", "seer", "witch"]
wolves, witch, seer = survivors[:2], survivors[-1], survivors[-2]
init中的model_config中的werewolf、witch、seer分别对应Player12、Player6、Player5,其余是villager
# night phase, werewolves discuss
hint = HostMsg(content=Prompts.to_wolves.format(n2s(wolves)))
with msghub(wolves, announcement=hint) as hub:
for _ in range(MAX_WEREWOLF_DISCUSSION_ROUND):
x = sequentialpipeline(wolves)
if x.get("agreement", False):
break
# werewolves vote
hint = HostMsg(content=Prompts.to_wolves_vote)
votes = [
extract_name_and_id(wolf(hint).content)[0] for wolf in wolves
]
# broadcast the result to werewolves
dead_player = [majority_vote(votes)]
hub.broadcast(
HostMsg(content=Prompts.to_wolves_res.format(dead_player[0])),
)
n2s(wolves)会将狼人的名称转换为一个格式化的字符串
msghub 为代理之间提供一种通信机制,使得每个代理可以发送消息,并且这些消息能够被其他所有代理接收
announcement作用是在 msghub 会话开始时向所有参与者广播一条消息,而不需要任何回应,在这是指挥狼人如何在夜晚进行行动和讨论
sequentialpipeline让狼人进行轮番询问,讨论结果
broadcast对于每个participant调用observe,observe调用memory.add,向_content中检查或添加记忆单元id,将embedding后的记忆单元放入memory_unit.embedding
# witch
healing_used_tonight = False
if witch in survivors:
if healing:
hint = HostMsg(
content=Prompts.to_witch_resurrect.format_map(
{
"witch_name": witch.name,
"dead_name": dead_player[0],
},
),
)
if witch(hint).get("resurrect", False):
healing_used_tonight = True
dead_player.pop()
healing = False
if poison and not healing_used_tonight:
x = witch(HostMsg(content=Prompts.to_witch_poison))
if x.get("eliminate", False):
dead_player.append(extract_name_and_id(x.content)[0])
poison = False
# seer
if seer in survivors:
hint = HostMsg(
content=Prompts.to_seer.format(seer.name, n2s(survivors)),
)
x = seer(hint)
player, idx = extract_name_and_id(x.content)
role = "werewolf" if roles[idx] == "werewolf" else "villager"
hint = HostMsg(content=Prompts.to_seer_result.format(player, role))
seer.observe(hint)
survivors, wolves = update_alive_players(
survivors,
wolves,
dead_player,
)
if check_winning(survivors, wolves, "Moderator"):
break
检查deadname,更新阵营
# daytime discussion
content = (
Prompts.to_all_danger.format(n2s(dead_player))
if dead_player
else Prompts.to_all_peace
)
hints = [
HostMsg(content=content),
HostMsg(content=Prompts.to_all_discuss.format(n2s(survivors))),
]
with msghub(survivors, announcement=hints) as hub:
# discuss
x = sequentialpipeline(survivors)
# vote
hint = HostMsg(content=Prompts.to_all_vote.format(n2s(survivors)))
votes = [
extract_name_and_id(_(hint).content)[0] for _ in survivors
]
vote_res = majority_vote(votes)
# broadcast the result to all players
result = HostMsg(content=Prompts.to_all_res.format(vote_res))
hub.broadcast(result)
survivors, wolves = update_alive_players(
survivors,
wolves,
vote_res,
)
if check_winning(survivors, wolves, "Moderator"):
break
hub.broadcast(HostMsg(content=Prompts.to_all_continue))
deadplayer为空就all_peace
hints提示白天讨论的流程
更多推荐
所有评论(0)