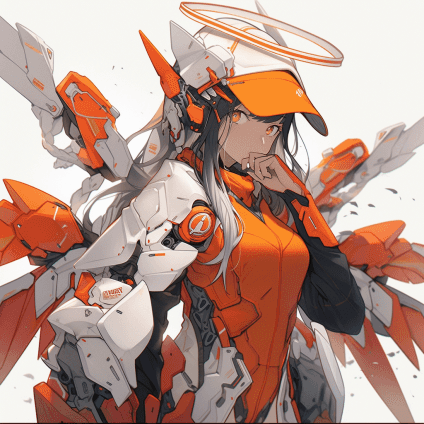
多Agent协作工具CrewAI使用指南——工具使用
默认情况下,该工具使用 OpenAI 进行嵌入和摘要生成。llm=dict(provider="ollama", # 或 google, openai, anthropic, llama2, ...# top_p=1,),),),),默认情况下,该工具同时使用 OpenAI 进行嵌入和摘要生成。llm=dict(provider="ollama", # 或 google, openai, anth
中文文档:http://www.aidoczh.com/crewai/
文章目录
安装
要使用文本搜索工具,您首先需要安装 crewai_tools 软件包。这可以通过 pip(Python 的软件包管理器)来完成。打开您的终端或命令提示符,输入以下命令:
pip install 'crewai[tools]'
这个命令将下载并安装文本搜索工具以及任何必要的依赖项。
文本搜索工具
描述
该工具用于在文本文件的内容中执行 RAG(检索增强生成)搜索。它允许在指定文本文件的内容中进行语义搜索,根据提供的查询快速提取信息或查找特定文本部分,是一个非常有价值的资源。
示例
以下示例演示了如何使用文本搜索工具在文本文件中进行搜索。该示例展示了如何初始化工具并在文件内容中进行搜索。
from crewai_tools import TXTSearchTool
# 初始化工具以在执行期间学习到的任何文本文件内容中进行搜索
tool = TXTSearchTool()
# 或者
# 使用特定文本文件初始化工具,以便代理程序可以在给定文本文件的内容中进行搜索
tool = TXTSearchTool(txt='path/to/text/file.txt')
参数
txt
(str): 可选。要搜索的文本文件的路径。只有在工具未使用特定文本文件进行初始化时才需要此参数;否则,搜索将在最初提供的文本文件中进行。
自定义模型和嵌入
默认情况下,该工具使用 OpenAI 进行嵌入和摘要生成。要自定义模型,您可以使用以下配置字典:
tool = TXTSearchTool(
config=dict(
llm=dict(
provider="ollama", # 或 google, openai, anthropic, llama2, ...
config=dict(
model="llama2",
# temperature=0.5,
# top_p=1,
# stream=true,
),
),
embedder=dict(
provider="google",
config=dict(
model="models/embedding-001",
task_type="retrieval_document",
# title="Embeddings",
),
),
)
)
PDFSearchTool
描述
PDFSearchTool 是一款专为在 PDF 内容中进行语义搜索而设计的 RAG 工具。它允许输入搜索查询和 PDF 文档,利用先进的搜索技术高效地找到相关内容。这种能力使其特别适用于快速从大型 PDF 文件中提取特定信息。
示例
以下是如何使用 PDFSearchTool 在 PDF 文档中进行搜索:
from crewai_tools import PDFSearchTool
# 初始化工具,允许在执行期间提供路径时进行任何 PDF 内容搜索
tool = PDFSearchTool()
# 或者
# 使用特定的 PDF 路径初始化工具,以在该文档中进行独占搜索
tool = PDFSearchTool(pdf='path/to/your/document.pdf')
参数
pdf
: 可选 用于搜索的 PDF 路径。可以在初始化时提供,也可以在run
方法的参数中提供。如果在初始化时提供,工具将限制其搜索范围为指定文档。
自定义模型和嵌入
默认情况下,该工具同时使用 OpenAI 进行嵌入和摘要生成。要自定义模型,可以使用以下配置字典:
tool = PDFSearchTool(
config=dict(
llm=dict(
provider="ollama", # 或 google, openai, anthropic, llama2, ...
config=dict(
model="llama2",
# temperature=0.5,
# top_p=1,
# stream=true,
),
),
embedder=dict(
provider="google",
config=dict(
model="models/embedding-001",
task_type="retrieval_document",
# title="Embeddings",
),
),
)
)
DOCXSearchTool
描述
DOCXSearchTool 是一款专为在 DOCX 文档中进行语义搜索而设计的 RAG 工具。它使用户能够通过基于查询的搜索有效地搜索和提取 DOCX 文件中的相关信息。该工具对于数据分析、信息管理和研究任务至关重要,简化了在大型文档集合中查找特定信息的过程。
示例
以下示例演示了如何初始化 DOCXSearchTool 来搜索任何 DOCX 文件的内容或特定 DOCX 文件路径中的内容。
from crewai_tools import DOCXSearchTool
# 初始化工具以搜索任何 DOCX 文件的内容
tool = DOCXSearchTool()
# 或者
# 使用特定的 DOCX 文件初始化工具,这样代理就只能搜索指定 DOCX 文件的内容
tool = DOCXSearchTool(docx='path/to/your/document.docx')
参数
docx
: 要搜索的特定 DOCX 文档的可选文件路径。如果在初始化期间未提供,该工具允许稍后指定任何 DOCX 文件的内容路径进行搜索。
自定义模型和嵌入
默认情况下,该工具在嵌入和摘要方面使用 OpenAI。要自定义模型,可以使用以下配置字典:
tool = DOCXSearchTool(
config=dict(
llm=dict(
provider="ollama", # 或 google, openai, anthropic, llama2, ...
config=dict(
model="llama2",
# temperature=0.5,
# top_p=1,
# stream=true,
),
),
embedder=dict(
provider="google",
config=dict(
model="models/embedding-001",
task_type="retrieval_document",
# title="Embeddings",
),
),
)
)
DirectoryReadTool
描述
DirectoryReadTool 是一个强大的实用工具,旨在提供目录内容的全面列表。它可以递归地浏览指定目录,为用户提供所有文件的详细枚举,包括子目录中的文件。这个工具对需要对目录结构进行彻底清点的任务或验证目录中文件组织的任务至关重要。
示例
使用 DirectoryReadTool 很简单。以下代码片段演示了如何设置并使用该工具来列出指定目录的内容:
from crewai_tools import DirectoryReadTool
# 初始化工具,以便代理程序在执行过程中了解任何目录的内容
tool = DirectoryReadTool()
# 或者
# 使用特定目录初始化工具,以便代理程序仅能读取指定目录的内容
tool = DirectoryReadTool(directory='/path/to/your/directory')
参数
DirectoryReadTool 需要进行最少配置以供使用。该工具的基本参数如下:
directory
:可选。指定要列出其内容的目录路径的参数。它接受绝对路径和相对路径,指导工具到达所需目录以进行内容列表。`
目录搜索工具
描述
目录搜索工具可以在指定目录的内容中进行语义搜索,利用检索增强生成(RAG)方法来实现对文件的高效导航。设计灵活,允许用户在运行时动态指定搜索目录,或在初始设置期间设置固定目录。
初始化和使用
从 crewai_tools
包中导入 DirectorySearchTool 来开始。您可以在不指定目录的情况下初始化工具,从而可以在运行时设置搜索目录。另外,也可以使用预定义目录初始化工具。
from crewai_tools import DirectorySearchTool
# 对于在运行时动态指定目录
tool = DirectorySearchTool()
# 对于固定目录搜索
tool = DirectorySearchTool(directory='/path/to/directory')
参数
directory
: 一个字符串参数,用于指定搜索目录。在初始化时是可选的,但如果最初未设置,则在搜索时是必需的。
自定义模型和嵌入
目录搜索工具默认使用 OpenAI 进行嵌入和摘要生成。这些设置的自定义选项包括更改模型提供商和配置,为高级用户增强了灵活性。
tool = DirectorySearchTool(
config=dict(
llm=dict(
provider="ollama", # 选项包括 ollama、google、anthropic、llama2 等
config=dict(
model="llama2",
# 这里添加额外的配置
),
),
embedder=dict(
provider="google",
config=dict(
model="models/embedding-001",
task_type="retrieval_document",
# title="Embeddings",
),
),
)
)
CSVSearchTool
描述
该工具用于在 CSV 文件内容中执行 RAG(检索增强生成)搜索。它允许用户在指定 CSV 文件的内容中语义搜索查询。这个功能特别适用于从大型 CSV 数据集中提取信息,传统搜索方法可能效率低下的情况。所有名称中带有“Search”的工具,包括 CSVSearchTool,在设计上都是用于搜索不同数据源的 RAG 工具。
示例
from crewai_tools import CSVSearchTool
# 使用特定的 CSV 文件初始化工具。这样设置允许代理只搜索给定的 CSV 文件。
tool = CSVSearchTool(csv='path/to/your/csvfile.csv')
# 或者
# 在没有特定 CSV 文件的情况下初始化工具。代理将需要在运行时提供 CSV 路径。
tool = CSVSearchTool()
参数
csv
:要搜索的 CSV 文件的路径。如果工具在没有特定 CSV 文件的情况下初始化,则这是一个必需的参数;否则,它是可选的。
自定义模型和嵌入
默认情况下,该工具同时使用 OpenAI 进行嵌入和摘要。要自定义模型,可以使用以下配置字典:
tool = CSVSearchTool(
config=dict(
llm=dict(
provider="ollama", # 或 google, openai, anthropic, llama2, ...
config=dict(
model="llama2",
# temperature=0.5,
# top_p=1,
# stream=true,
),
),
embedder=dict(
provider="google",
config=dict(
model="models/embedding-001",
task_type="retrieval_document",
# title="Embeddings",
),
),
)
)
文件读取工具
描述
文件读取工具在 crewai_tools 软件包中概念上代表了一套旨在促进文件读取和内容检索的功能。该套件包括用于处理批量文本文件、读取运行时配置文件以及导入分析数据的工具。它支持多种基于文本的文件格式,如 .txt
、.csv
、.json
等。根据文件类型,该套件提供了专门的功能,例如将 JSON 内容转换为 Python 字典以便于使用。
使用示例
要开始使用文件读取工具:
from crewai_tools import FileReadTool
# 初始化工具以读取代理已知的任何文件或学习指定文件的路径
file_read_tool = FileReadTool()
# 或者
# 使用特定文件路径初始化工具,这样代理只能读取指定文件的内容
file_read_tool = FileReadTool(file_path='path/to/your/file.txt')
参数
file_path
: 您要读取的文件路径。它接受绝对路径和相对路径。确保文件存在并且您具有访问权限。
MDXSearchTool
描述
MDXSearchTool 是 crewai_tools
软件包的一个组件,旨在促进高级市场数据提取。对于寻求快速获取市场见解的研究人员和分析师来说,这个工具非常宝贵,尤其是在人工智能领域。它通过与各种数据源进行接口,简化了获取、解释和组织市场数据的任务。
使用示例
要使用 MDXSearchTool,您必须首先设置必要的环境变量。然后,将该工具集成到您的 crewAI 项目中,开始您的市场研究。以下是如何执行此操作的基本示例:
from crewai_tools import MDXSearchTool
# 初始化工具以搜索执行过程中了解到的任何 MDX 内容
tool = MDXSearchTool()
# 或者
# 使用特定的 MDX 文件路径初始化工具,以在该文档中进行独占搜索
tool = MDXSearchTool(mdx='path/to/your/document.mdx')
参数
- mdx: 可选。指定搜索的 MDX 文件路径。可以在初始化过程中提供。
模型和嵌入的定制化
该工具默认使用 OpenAI 进行嵌入和摘要生成。要进行定制化,可以使用如下所示的配置字典:
tool = MDXSearchTool(
config=dict(
llm=dict(
provider="ollama", # 选项包括 google, openai, anthropic, llama2 等。
config=dict(
model="llama2",
# 可在此处包括可选参数。
# temperature=0.5,
# top_p=1,
# stream=true,
),
),
embedder=dict(
provider="google",
config=dict(
model="models/embedding-001",
task_type="retrieval_document",
# 可在此处添加嵌入的可选标题。
# title="Embeddings",
),
),
)
)
网页抓取工具
描述
这是一个旨在提取和阅读指定网站内容的工具。它能够通过发出 HTTP 请求并解析接收到的 HTML 内容来处理各种类型的网页。该工具特别适用于网络抓取任务、数据收集或从网站中提取特定信息。
示例
from crewai_tools import ScrapeWebsiteTool
# 若要在执行期间启用对任何找到的网站进行抓取
tool = ScrapeWebsiteTool()
# 使用网站 URL 初始化工具,以便代理只能抓取指定网站的内容
tool = ScrapeWebsiteTool(website_url='https://www.example.com')
# 从网站提取文本
text = tool.run()
print(text)
参数
website_url
:必填的网站 URL 以读取文件。这是工具的主要输入,指定应抓取和阅读哪个网站的内容。
网站搜索工具
描述
网站搜索工具旨在作为在网站内容中进行语义搜索的概念设计。它旨在利用像检索增强生成(RAG)这样的先进机器学习模型,以高效地浏览和提取指定 URL 中的信息。该工具旨在提供灵活性,允许用户在任何网站上执行搜索或专注于感兴趣的特定网站。请注意,网站搜索工具的当前实现细节正在开发中,所述功能可能尚不可访问。
示例用法
以下是网站搜索工具在不同场景下的使用示例。请注意,这些示例是说明性的,代表了计划功能:
from crewai_tools import WebsiteSearchTool
# 初始化工具,代理可以使用它在任何发现的网站上进行搜索
tool = WebsiteSearchTool()
# 限制搜索范围为特定网站的内容,现在代理只能在该网站内进行搜索
tool = WebsiteSearchTool(website='https://example.com')
参数
website
:一个可选参数,用于指定用于重点搜索的网站 URL。此参数旨在通过在必要时允许定向搜索来增强工具的灵活性。
自定义选项
默认情况下,该工具在嵌入和摘要生成方面使用 OpenAI。要自定义模型,您可以使用以下配置字典:
tool = WebsiteSearchTool(
config=dict(
llm=dict(
provider="ollama", # 或 google, openai, anthropic, llama2, ...
config=dict(
model="llama2",
# temperature=0.5,
# top_p=1,
# stream=true,
),
),
embedder=dict(
provider="google",
config=dict(
model="models/embedding-001",
task_type="retrieval_document",
# title="Embeddings",
),
),
)
)
SeleniumScrapingTool
描述
SeleniumScrapingTool 专为高效的网络抓取任务而设计。它通过使用 CSS 选择器来精确提取网页内容,满足各种抓取需求,灵活处理任何提供的网站 URL。
使用示例
以下是 SeleniumScrapingTool 可以使用的一些场景:
from crewai_tools import SeleniumScrapingTool
# 示例 1:初始化工具,无需任何参数即可抓取当前导航到的页面
tool = SeleniumScrapingTool()
# 示例 2:抓取给定 URL 的整个网页
tool = SeleniumScrapingTool(website_url='https://example.com')
# 示例 3:从网页中定位并抓取特定的 CSS 元素
tool = SeleniumScrapingTool(website_url='https://example.com', css_element='.main-content')
# 示例 4:使用附加参数执行抓取,获得定制化体验
tool = SeleniumScrapingTool(website_url='https://example.com', css_element='.main-content', cookie={'name': 'user', 'value': 'John Doe'}, wait_time=10)
参数
以下参数可用于自定义 SeleniumScrapingTool 的抓取过程:
website_url
:必填。指定要从中抓取内容的网站 URL。css_element
:必填。要在网站上定位的特定元素的 CSS 选择器。这使得可以集中抓取网页的特定部分。cookie
:可选。包含 cookie 信息的字典。用于模拟已登录会话,从而提供对可能仅限于未登录用户的内容的访问。wait_time
:可选。指定在抓取内容之前的延迟时间(以秒为单位)。此延迟确保网站和任何动态内容完全加载,以确保成功抓取。
PGSearchTool
描述
PGSearchTool 被设想为一个强大的工具,用于在 PostgreSQL 数据库表中进行语义搜索。通过利用先进的检索和生成(RAG)技术,它旨在提供一种有效的方式来查询数据库表内容,专门为 PostgreSQL 数据库定制。该工具的目标是通过语义搜索查询简化查找相关数据的过程,为需要在 PostgreSQL 环境中对大型数据集进行高级查询的用户提供宝贵资源。
示例用法
以下是一个示例,展示如何使用 PGSearchTool 在 PostgreSQL 数据库中的表上进行语义搜索:
from crewai_tools import PGSearchTool
# 使用数据库 URI 和目标表名初始化工具
tool = PGSearchTool(db_uri='postgresql://user:password@localhost:5432/mydatabase', table_name='employees')
参数
PGSearchTool 设计为需要以下参数才能运行:
db_uri
:表示要查询的 PostgreSQL 数据库的 URI 的字符串。此参数将是必需的,必须包括必要的身份验证详细信息和数据库的位置。table_name
:指定将在其中执行语义搜索的数据库中表的名称的字符串。此参数也将是必需的。
自定义模型和嵌入
该工具打算默认使用 OpenAI 进行嵌入和摘要。用户将可以使用以下配置字典自定义模型:
tool = PGSearchTool(
config=dict(
llm=dict(
provider="ollama", # 或 google, openai, anthropic, llama2, ...
config=dict(
model="llama2",
# temperature=0.5,
# top_p=1,
# stream=true,
),
),
embedder=dict(
provider="google",
config=dict(
model="models/embedding-001",
task_type="retrieval_document",
# title="Embeddings",
),
),
)
)
更多推荐
所有评论(0)